Revolutionizing AI Safety: OpenAI's Innovative Red Teaming Techniques
- School of AI
- Nov 28, 2024
- 2 min read
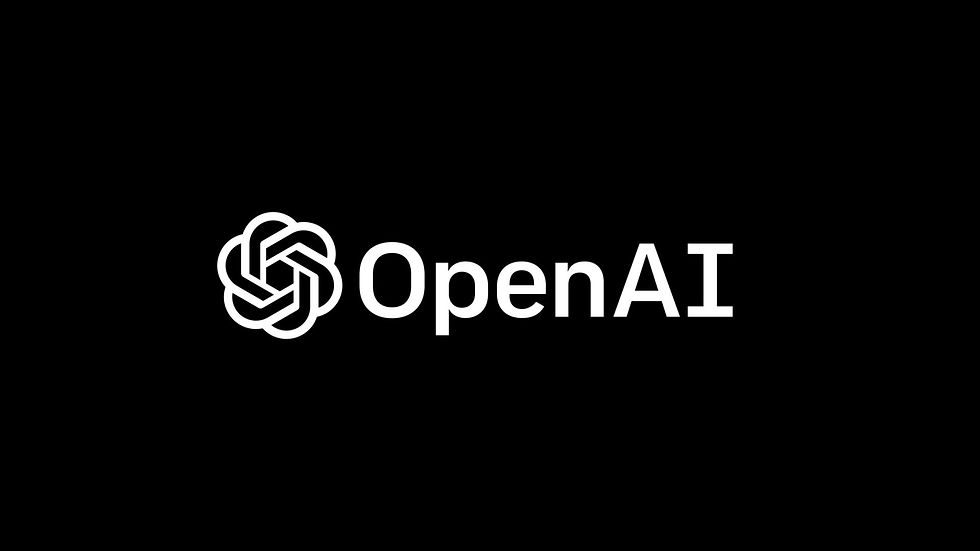
A cornerstone of OpenAI’s commitment to AI safety is its adoption of red teaming—a structured approach that uses human expertise and AI tools to uncover and address risks in its models.
In 2022, OpenAI utilized manual testing to rigorously assess vulnerabilities in the DALL·E 2 image generation model. Since then, its methodologies have evolved, incorporating automated and hybrid approaches to create a more robust framework for risk evaluation.
As OpenAI stated, “Our goal is to harness more powerful AI to identify and correct model errors at scale.” This vision drives their continuous innovation in red teaming techniques.

Advancing Red Teaming: New Tools & Research
OpenAI’s recent initiatives include two key releases:
A white paper detailing strategies for engaging external experts in red teaming.
A research study introducing automated methods that enhance efficiency and diversity in identifying potential risks.
These advancements aim to refine red teaming processes, ensuring safer and more responsible AI systems.

Why Red Teaming is Essential
As AI systems grow in complexity, addressing risks like abuse and misuse is crucial. By blending insights from external experts with systematic testing, red teaming helps establish safety benchmarks and align AI systems with societal values.

The Four-Step Approach to Human-Centered Red Teaming
In the white paper, “OpenAI’s Approach to External Red Teaming for AI Models and Systems,” OpenAI outlines its four-step framework:
Selecting Diverse Teams: Experts from various fields, such as natural sciences, cybersecurity, and geopolitics, bring comprehensive perspectives.
Access to Model Versions: Teams analyze vulnerabilities in early-stage models and assess safety measures in advanced iterations.
Guidance and Documentation: Structured reporting and intuitive interfaces facilitate effective testing and documentation.
Data Synthesis and Evaluation: Post-campaign insights drive continuous improvements in safety protocols.
This approach was recently implemented to prepare OpenAI’s o1 family of models for public use, testing their resilience against misuse across diverse domains.

Automation in Red Teaming
OpenAI’s latest research introduces “Diverse and Effective Red Teaming with Auto-Generated Rewards and Multi-Step Reinforcement Learning.” This method enhances the diversity and effectiveness of automated attack strategies, enabling models to simulate scenarios such as illicit advice and train against these risks.
Addressing Challenges
While red teaming captures risks at a specific moment, evolving AI models and potential information hazards pose ongoing challenges. OpenAI mitigates these risks through responsible disclosure and public engagement to shape AI policies that align with ethical standards.
With its innovative red teaming approaches, OpenAI is setting new benchmarks for AI safety and advancing its mission of creating safer, more reliable systems.
API Connects is a global IT services firm in New Zealand brand excelling in Technology Architecture, Consulting, Software development & DevOps. Consult today! Visit: https://apiconnects.co.nz/devops-infrastructure-management/